
The strategic approach to Generative AI in enterprise products
Pablo Romeo
Co-Founder & CTO
In 2025, integrating AI into enterprise products is a major market differentiator for businesses. However, developing a generative product demands a tailored approach to ensure effective implementation and maximize resource investment.
At CloudX, we specialize in embedding Generative AI into enterprise solutions, enhancing functionality and driving growth. For us, tackling AI projects requires a strategic, structured approach that differs significantly from traditional development methodologies. We’ve developed a comprehensive framework grounded in a deep understanding of AI's capabilities and its practical applications. This methodology guides our clients through AI projects with precision and clarity, ensuring that their investments lead to measurable improvements in efficiency and growth.
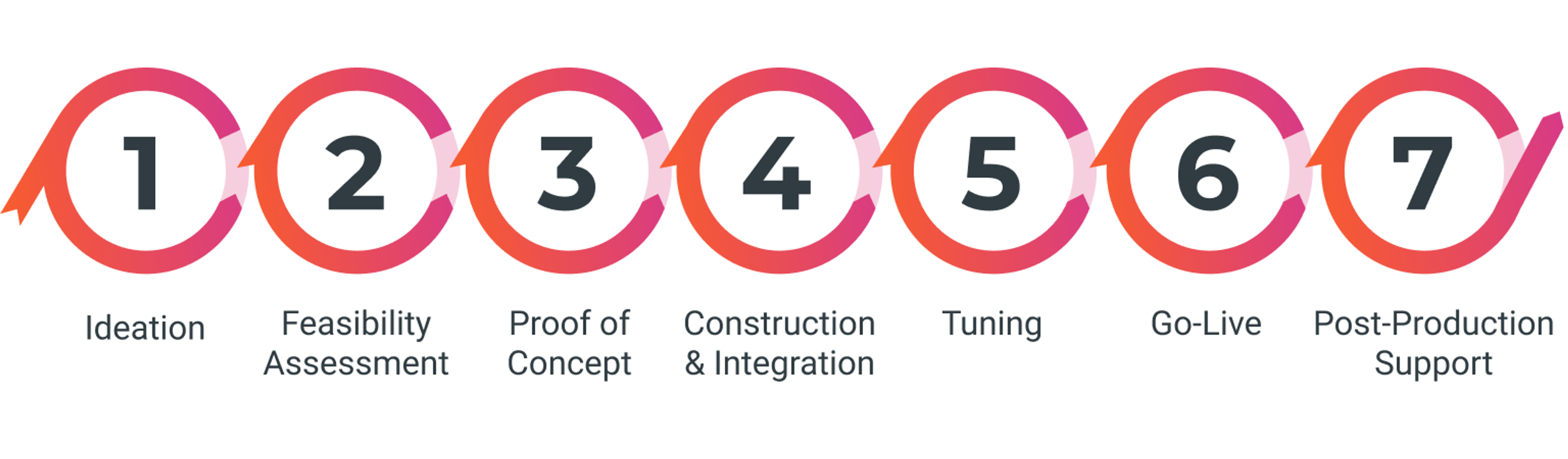
Getting started: the Planning Phase
Our approach to Generative AI projects begins with meticulous planning, a critical phase that establishes the foundation for developing a successful enterprise product. This ensures alignment with our client's strategic business goals. Our objective is not merely to implement AI for its own sake, but to ensure its integration makes sense and enhances business processes, optimizing productivity. Each company has distinct business requirements, so each solution must be tailored to fit seamlessly.
Step 1: Ideation
The journey begins with Ideation, where we brainstorm innovative solutions to help our clients realize their vision. Unlike traditional development, which often addresses predefined problems, our approach to Generative AI projects involves a collaborative visioning process. We work closely with our client's team to identify unique business needs, envisioning how AI can be meaningfully integrated into their existing ecosystem. The Ideation stage ensures that AI initiatives align with business objectives, setting the stage for solutions that are both technologically advanced and operationally effective.
Step 2: Feasibility Assessment
Following the visioning process, we conduct a comprehensive feasibility assessment. Traditional development often focuses solely on technical feasibility, but our approach is holistic. We evaluate usability, scalability, security, data privacy, and operational costs—among other aspects—, ensuring a thorough analysis. This phase involves analyzing various AI models and agentic frameworks to determine the best fit for our client's needs.
The objective of the Feasibility Assessment is to find out whether the envisioned solution is achievable and if the investment aligns with the desired outcomes. While some challenges may not be solvable with current technology, our focus is on creating solutions that improve as the underlying technology advances. For instance, if the Large Language Models (LLMs) selected for our project improve over time, the product as a whole benefits as well. This approach ensures our solutions can grow and adapt alongside the business, providing long-term value and enhancing their effectiveness as technology evolves.
Step 3: Proof of Concept
In this stage, we develop prototypes to validate the tech stack, architecture, and external services to be used in the solution. Our goal is to identify the most effective approach to solving our client's problem. The Proof of Concept is key for demonstrating which agentic frameworks and LLMs truly deliver results, laying the foundation upon which we build the final product.
During this phase, we explore several critical questions, including:
🔸 Is this agent suitable for addressing our client's specific needs?
🔸 What is the optimal implementation of the agent for this scenario?
🔸 How does the proposed solution integrate with existing systems and processes?
🔸 What are the potential scalability challenges, and how can they be mitigated?
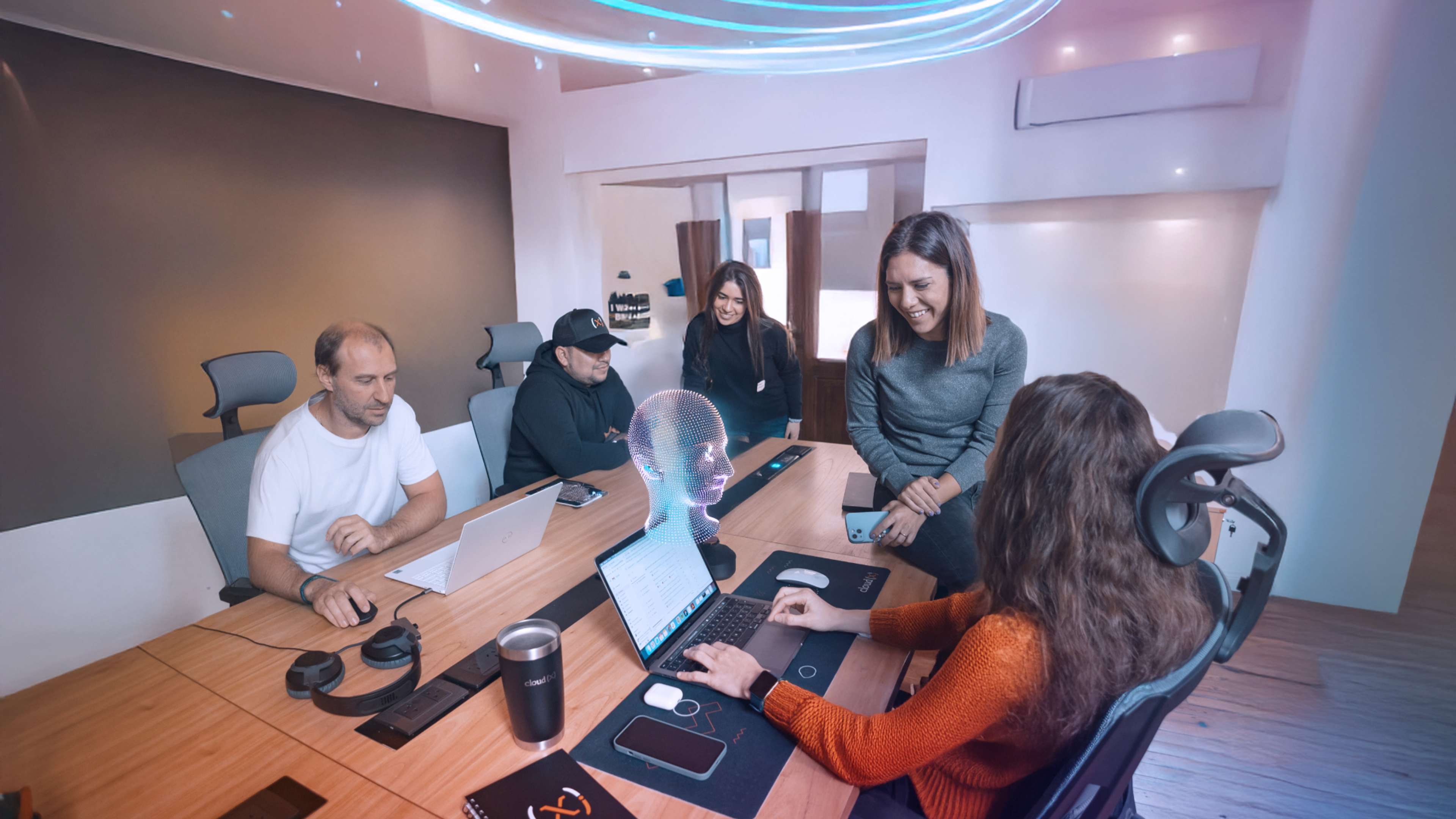
Hands-on: the Implementation Phase
With the Planning Phase complete, we transition to building the enterprise product. The Implementation Phase is where strategic planning translates into tangible results.
Step 4: Construction & Integration
This phase is where the magic happens. Our team builds and integrates the AI software to function as an integral part of our client’s business infrastructure. Unlike traditional development, this process involves unique tooling, such as LLMs, agentic frameworks, and specialized observability tools.
Building a generative product is inherently more iterative than traditional development. The initial implementation may work adequately, but it is through continuous improvement over time that it truly excels. Construction and enhancement occur in an iterative cycle, where the optimal approach to prompting, tool selection, and cost estimation cannot be precisely predetermined. This iterative nature introduces a higher degree of uncertainty, necessitating flexibility and adaptability.
A key distinction in AI application development is the testing approach. Traditional testing methods are complemented by AI-specific evaluations, known as evals. These involve creating datasets based on expected inputs and outputs, using generative technology to validate the results. Here, the concept of LLM as a judge comes into play, where the LLM evaluates the conversation, input, information used, and the outcome.
Step 5: Tuning
Once the software solution is built, we focus on refining the model's usage through techniques like prompt engineering, RAG, tool augmentation, and fine-tuning. This continuous optimization approach allows us to monitor behavior and operational parameters, ensuring that the AI solution delivers quantifiable improvements in operational efficiency.
Agentic solutions, being non-deterministic, require a statistical review. The development process combines traditional methods with Generative AI development, validated through LLMOps and observability—tracing, input/output, costs, among others. This information can also be cross-referenced with user feedback for a comprehensive understanding of how the solution performs, enabling us to fine-tune it for excellence.
Observability tools and cost control measures play a crucial role in this phase. For instance, if a solution performs well but incurs high costs, we can adjust by using more advanced models for critical functions and more cost-effective models for less demanding tasks. Our goal is to optimize both costs and outcomes, ensuring the solution adapts to evolving needs and market conditions.
Step 6: Go-Live
The go-live phase is a critical milestone in any project, and our approach ensures a seamless roll-out. By working closely with our client's organization, we minimize risk and maximize return on investment, ensuring that the transition to AI-powered operations is smooth and minimally disruptive. Our comprehensive support during this phase ensures that the AI solution is fully integrated and operational from day one.
In the realm of Generative AI, the journey from a basic generative product to a production-grade enterprise solution is challenging. The initial 50% of development may seem straightforward, but each subsequent increment becomes increasingly complex. The industry can be misleading, as creating a basic demo is relatively easy, but developing a predictable, consistent, and reliable enterprise solution that avoids issues like hallucinations is far more demanding.
Step 7: Post-Production Support
Support doesn’t end with deployment. Our post-production support continues until the project is fully operational and expected standards are met. It is critical to perform periodic reviews of usability and collected data for continuous improvement of the user experience. This approach ensures long-term success, turning AI adoption into a sustainable competitive advantage for our client.
In this phase, the solution is exposed to the world, and we focus on iteratively improving the product based on user feedback and usage metrics. The AI solution doesn't learn on its own; it requires deliberate enhancements informed by what worked well and what didn't. By leveraging insights from user interactions and operational data, we ensure the AI solution remains aligned with our client's strategic objectives.
Bridging Generative AI and enterprise transformation
Building a generative product is a pivotal step in the broader macro transformation of an enterprise through AI. This process is not just about developing a technological solution but about thoughtfully integrating AI to boost business operations. A well-defined plan, strategy, and process are crucial to navigating the complexities of Cognitive Transformation, helping to minimize risks and optimize resources.
As AI increasingly becomes a key factor for competitive advantage, we hope the insights shared in this article offer a useful roadmap for enterprises looking to explore the full spectrum of AI capabilities. This discussion aims to set the stage for further exploration into how businesses can continue to innovate and adapt. The journey of AI transformation is ongoing, and there is much more to learn about the strategies that can empower organizations to thrive in the AI era.
Related Content
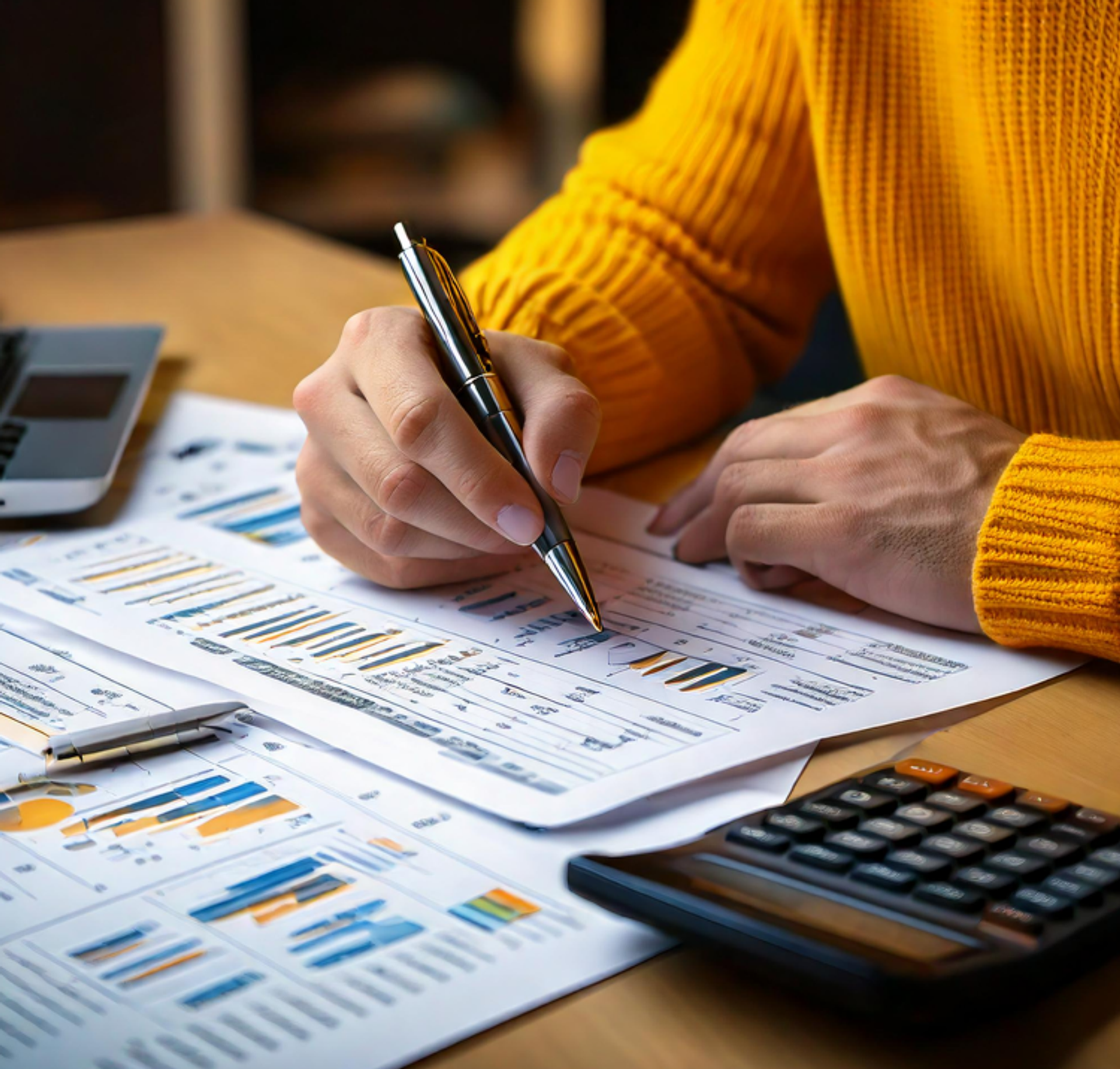
Success Case
Transforming Financial Data Extraction With AI for a Global Professional Services Firm
Leveraging Generative AI, we automated the extraction of data from 3,000 to 7,000 financial documents yearly for a top professional services firm, saving…
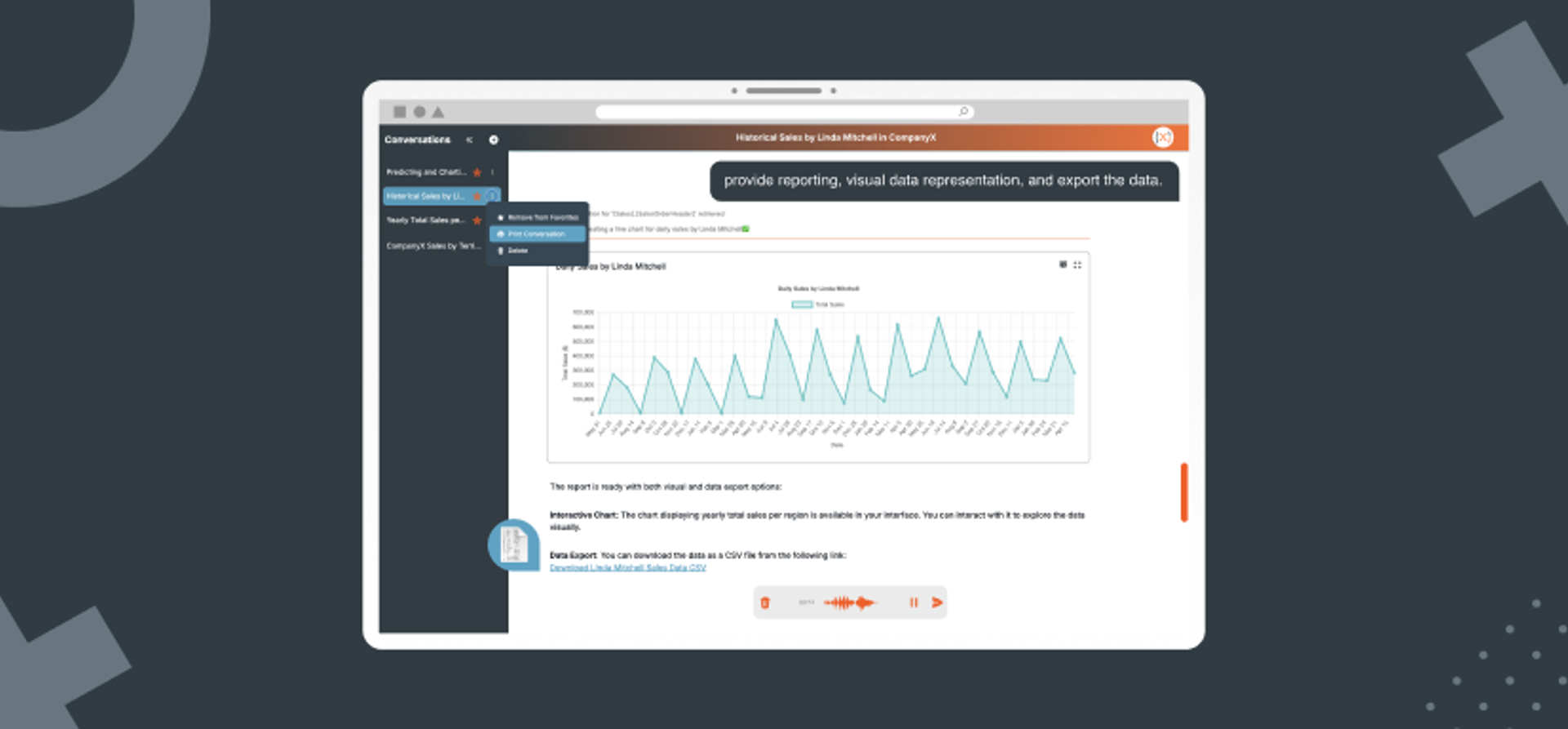
Enterprise AI Solution
Talk to Database
A versatile, extensible, and customizable AI Agent designed to transform the way you interact with your business data.
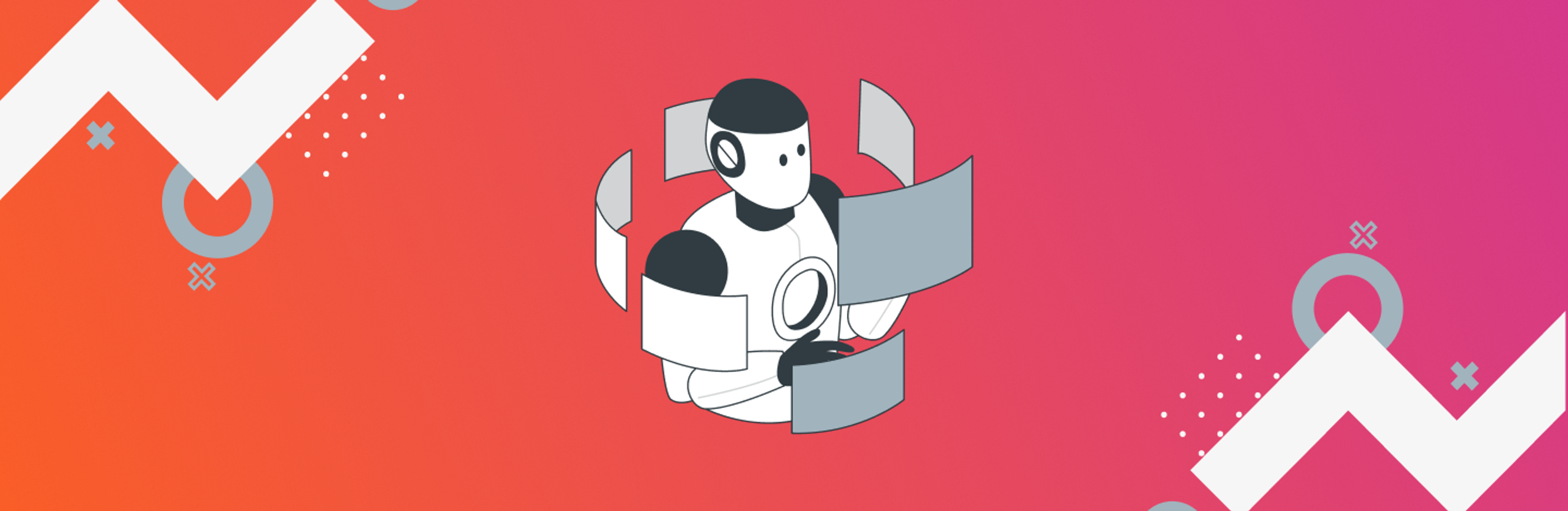
Blog Article
AI Agent Experience: the current UX paradigm is about to change
UX for digital products and services is evolving as AI Agents emerge as new consumers. With advancements in Generative AI, humans increasingly delegate tasks…